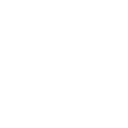
Go offline with the Player FM app!
Reasoning, Robustness, and Human Feedback in AI - Max Bartolo (Cohere)
Manage episode 472105428 series 2803422
Dr. Max Bartolo from Cohere discusses machine learning model development, evaluation, and robustness. Key topics include model reasoning, the DynaBench platform for dynamic benchmarking, data-centric AI development, model training challenges, and the limitations of human feedback mechanisms. The conversation also covers technical aspects like influence functions, model quantization, and the PRISM project.
Max Bartolo (Cohere):
https://www.maxbartolo.com/
https://cohere.com/command
TRANSCRIPT:
https://www.dropbox.com/scl/fi/vujxscaffw37pqgb6hpie/MAXB.pdf?rlkey=0oqjxs5u49eqa2m7uaol64lbw&dl=0
TOC:
1. Model Reasoning and Verification
[00:00:00] 1.1 Model Consistency and Reasoning Verification
[00:03:25] 1.2 Influence Functions and Distributed Knowledge Analysis
[00:10:28] 1.3 AI Application Development and Model Deployment
[00:14:24] 1.4 AI Alignment and Human Feedback Limitations
2. Evaluation and Bias Assessment
[00:20:15] 2.1 Human Evaluation Challenges and Factuality Assessment
[00:27:15] 2.2 Cultural and Demographic Influences on Model Behavior
[00:32:43] 2.3 Adversarial Examples and Model Robustness
3. Benchmarking Systems and Methods
[00:41:54] 3.1 DynaBench and Dynamic Benchmarking Approaches
[00:50:02] 3.2 Benchmarking Challenges and Alternative Metrics
[00:50:33] 3.3 Evolution of Model Benchmarking Methods
[00:51:15] 3.4 Hierarchical Capability Testing Framework
[00:52:35] 3.5 Benchmark Platforms and Tools
4. Model Architecture and Performance
[00:55:15] 4.1 Cohere's Model Development Process
[01:00:26] 4.2 Model Quantization and Performance Evaluation
[01:05:18] 4.3 Reasoning Capabilities and Benchmark Standards
[01:08:27] 4.4 Training Progression and Technical Challenges
5. Future Directions and Challenges
[01:13:48] 5.1 Context Window Evolution and Trade-offs
[01:22:47] 5.2 Enterprise Applications and Future Challenges
REFS:
[00:03:10] Research at Cohere with Laura Ruis et al., Max Bartolo, Laura Ruis et al.
https://cohere.com/research/papers/procedural-knowledge-in-pretraining-drives-reasoning-in-large-language-models-2024-11-20
[00:04:15] Influence functions in machine learning, Koh & Liang
https://arxiv.org/abs/1703.04730
[00:08:05] Studying Large Language Model Generalization with Influence Functions, Roger Grosse et al.
https://storage.prod.researchhub.com/uploads/papers/2023/08/08/2308.03296.pdf
[00:11:10] The LLM ARChitect: Solving ARC-AGI Is A Matter of Perspective, Daniel Franzen, Jan Disselhoff, and David Hartmann
https://github.com/da-fr/arc-prize-2024/blob/main/the_architects.pdf
[00:12:10] Hugging Face model repo for C4AI Command A, Cohere and Cohere For AI
https://huggingface.co/CohereForAI/c4ai-command-a-03-2025
[00:13:30] OpenInterpreter
https://github.com/KillianLucas/open-interpreter
[00:16:15] Human Feedback is not Gold Standard, Tom Hosking, Max Bartolo, Phil Blunsom
https://arxiv.org/abs/2309.16349
[00:27:15] The PRISM Alignment Dataset, Hannah Kirk et al.
https://arxiv.org/abs/2404.16019
[00:32:50] How adversarial examples arise, Andrew Ilyas, Shibani Santurkar, Dimitris Tsipras, Logan Engstrom, Brandon Tran, Aleksander Madry
https://arxiv.org/abs/1905.02175
[00:43:00] DynaBench platform paper, Douwe Kiela et al.
https://aclanthology.org/2021.naacl-main.324.pdf
[00:50:15] Sara Hooker's work on compute limitations, Sara Hooker
https://arxiv.org/html/2407.05694v1
[00:53:25] DataPerf: Community-led benchmark suite, Mazumder et al.
https://arxiv.org/abs/2207.10062
[01:04:35] DROP, Dheeru Dua et al.
https://arxiv.org/abs/1903.00161
[01:07:05] GSM8k, Cobbe et al.
https://paperswithcode.com/sota/arithmetic-reasoning-on-gsm8k
[01:09:30] ARC, François Chollet
https://github.com/fchollet/ARC-AGI
[01:15:50] Command A, Cohere
https://cohere.com/blog/command-a
[01:22:55] Enterprise search using LLMs, Cohere
https://cohere.com/blog/commonly-asked-questions-about-search-from-coheres-enterprise-customers
217 episodes
Manage episode 472105428 series 2803422
Dr. Max Bartolo from Cohere discusses machine learning model development, evaluation, and robustness. Key topics include model reasoning, the DynaBench platform for dynamic benchmarking, data-centric AI development, model training challenges, and the limitations of human feedback mechanisms. The conversation also covers technical aspects like influence functions, model quantization, and the PRISM project.
Max Bartolo (Cohere):
https://www.maxbartolo.com/
https://cohere.com/command
TRANSCRIPT:
https://www.dropbox.com/scl/fi/vujxscaffw37pqgb6hpie/MAXB.pdf?rlkey=0oqjxs5u49eqa2m7uaol64lbw&dl=0
TOC:
1. Model Reasoning and Verification
[00:00:00] 1.1 Model Consistency and Reasoning Verification
[00:03:25] 1.2 Influence Functions and Distributed Knowledge Analysis
[00:10:28] 1.3 AI Application Development and Model Deployment
[00:14:24] 1.4 AI Alignment and Human Feedback Limitations
2. Evaluation and Bias Assessment
[00:20:15] 2.1 Human Evaluation Challenges and Factuality Assessment
[00:27:15] 2.2 Cultural and Demographic Influences on Model Behavior
[00:32:43] 2.3 Adversarial Examples and Model Robustness
3. Benchmarking Systems and Methods
[00:41:54] 3.1 DynaBench and Dynamic Benchmarking Approaches
[00:50:02] 3.2 Benchmarking Challenges and Alternative Metrics
[00:50:33] 3.3 Evolution of Model Benchmarking Methods
[00:51:15] 3.4 Hierarchical Capability Testing Framework
[00:52:35] 3.5 Benchmark Platforms and Tools
4. Model Architecture and Performance
[00:55:15] 4.1 Cohere's Model Development Process
[01:00:26] 4.2 Model Quantization and Performance Evaluation
[01:05:18] 4.3 Reasoning Capabilities and Benchmark Standards
[01:08:27] 4.4 Training Progression and Technical Challenges
5. Future Directions and Challenges
[01:13:48] 5.1 Context Window Evolution and Trade-offs
[01:22:47] 5.2 Enterprise Applications and Future Challenges
REFS:
[00:03:10] Research at Cohere with Laura Ruis et al., Max Bartolo, Laura Ruis et al.
https://cohere.com/research/papers/procedural-knowledge-in-pretraining-drives-reasoning-in-large-language-models-2024-11-20
[00:04:15] Influence functions in machine learning, Koh & Liang
https://arxiv.org/abs/1703.04730
[00:08:05] Studying Large Language Model Generalization with Influence Functions, Roger Grosse et al.
https://storage.prod.researchhub.com/uploads/papers/2023/08/08/2308.03296.pdf
[00:11:10] The LLM ARChitect: Solving ARC-AGI Is A Matter of Perspective, Daniel Franzen, Jan Disselhoff, and David Hartmann
https://github.com/da-fr/arc-prize-2024/blob/main/the_architects.pdf
[00:12:10] Hugging Face model repo for C4AI Command A, Cohere and Cohere For AI
https://huggingface.co/CohereForAI/c4ai-command-a-03-2025
[00:13:30] OpenInterpreter
https://github.com/KillianLucas/open-interpreter
[00:16:15] Human Feedback is not Gold Standard, Tom Hosking, Max Bartolo, Phil Blunsom
https://arxiv.org/abs/2309.16349
[00:27:15] The PRISM Alignment Dataset, Hannah Kirk et al.
https://arxiv.org/abs/2404.16019
[00:32:50] How adversarial examples arise, Andrew Ilyas, Shibani Santurkar, Dimitris Tsipras, Logan Engstrom, Brandon Tran, Aleksander Madry
https://arxiv.org/abs/1905.02175
[00:43:00] DynaBench platform paper, Douwe Kiela et al.
https://aclanthology.org/2021.naacl-main.324.pdf
[00:50:15] Sara Hooker's work on compute limitations, Sara Hooker
https://arxiv.org/html/2407.05694v1
[00:53:25] DataPerf: Community-led benchmark suite, Mazumder et al.
https://arxiv.org/abs/2207.10062
[01:04:35] DROP, Dheeru Dua et al.
https://arxiv.org/abs/1903.00161
[01:07:05] GSM8k, Cobbe et al.
https://paperswithcode.com/sota/arithmetic-reasoning-on-gsm8k
[01:09:30] ARC, François Chollet
https://github.com/fchollet/ARC-AGI
[01:15:50] Command A, Cohere
https://cohere.com/blog/command-a
[01:22:55] Enterprise search using LLMs, Cohere
https://cohere.com/blog/commonly-asked-questions-about-search-from-coheres-enterprise-customers
217 episodes
All episodes
×Welcome to Player FM!
Player FM is scanning the web for high-quality podcasts for you to enjoy right now. It's the best podcast app and works on Android, iPhone, and the web. Signup to sync subscriptions across devices.